by Xinbo Geng (Texas A&M University), Swati Gupta (Massachusetts Institute of Technology), Tong Huang (Texas A&M University), and Le Xie (Texas A&M University)
The electric grid serves as one of the backbone infrastructure systems that support the well-being of billions of citizens in modern society. There has been a significant transformation of energy systems over the past decade, even though these changes have largely gone unnoticed due to affordable and reliable grid services for end-users in most developed nations. The transformation on the supply side mainly manifests itself through the decarbonization of the power supply, i.e. greater use of low-carbon energy sources. As an example, a recent National Bureau of Economic Research (NBER) study released in December 2018 suggested that US power-sector emissions have decreased by 45% since 2008. This dramatic change is fundamentally driven by two factors: (1) a sharp increase in the renewable-energy portfolio; and (2) the substantial replacement of coal-fired power plants with natural gas and other resources.
Due to the supply-side transformation, in order to maintain or improve the quality of electricity services to end-users, it is necessary to make use of real-time information that is becoming readily available thanks to the proliferation of information and communication technology (ICT) infrastructures.
Decision making in the electric grid enabled by real-time information occurs at multiple time scales, which makes the problem even more interesting. At the time scale of 15 minutes or longer, a central challenge lies in how to best allocate different resources that will meet the balance of the fluctuating demand at the lowest cost. The underlying mathematical problem is typically formulated as a nonlinear multi-stage optimization problem. Another big area of investment in real-time decision making (RTDM) is in the proliferation of globally synchronized sensors called synchrophasors. Compared with conventional SCADA systems, synchrophasors offer a two-orders-of-magnitude faster sampling rate, and synchronized time stamps for electrical variables. Therefore, they offer new opportunities to detect, locate, and classify anomalies that would have not been possible to capture by conventional means, as well as opportunities to avoid blackouts.
In what follows, we elaborate on the real-time decision-making challenges and opportunities at two different time scales and two different spatial scales. The first one deals with day-ahead decisions on phase balancing in the distribution grid. The second one studies the possibility of real-time localization of anomalies such as forced oscillation in large transmission systems.
A focal area of the Spring 2018 Simons Institute program on Real-Time Decision Making was formalizing and studying various issues in the modern energy grid pertaining to efficiency, reliability and security. Among the research themes, a good amount of effort was devoted to modernizing the optimization core of grid operations [4]. In addition, by tapping into expertise in the program in combinatorial algorithms, concrete collaboration occurred at the interface between combinatorial algorithms and grid optimization. For example, electricity is typically distributed radially to address security concerns, and this translates to selecting a spanning tree in a network with desirable properties. The project below on the distribution grid grew out of frequent conversations that the RTDM program facilitated for researchers with backgrounds in optimization and in power-systems engineering.
Phase Balancing in the Electric Distribution Grid
The distribution system is a part of a power system after the transmission system, and is dedicated to delivering electric energy to end-users. All end-users are connected to one or two of three phases (A, B and C) of distribution systems. Phases are balanced if the total load on each phase is (roughly) the same, and it is a fundamental assumption in distribution system operations that the three phases are balanced. Unbalanced phases could lead to higher risks of equipment failures, increased delivery losses, potential relay malfunctioning, additional asset reinforcement costs, and issues related to voltage imbalances. For example, when a distribution system is unbalanced, phase A might reach its full capacity while the other two phases are significantly under-utilized, which is not cost efficient.
(source: https://en.wikipedia.org/wiki/Electric_power_transmission)
The phase-balancing problem aims to find the most balanced assignment of loads to three phases (A, B or C). A typical form of the phase-balancing problem minimizes the differences between a single-phase load and the ideal case (one-third of total load).
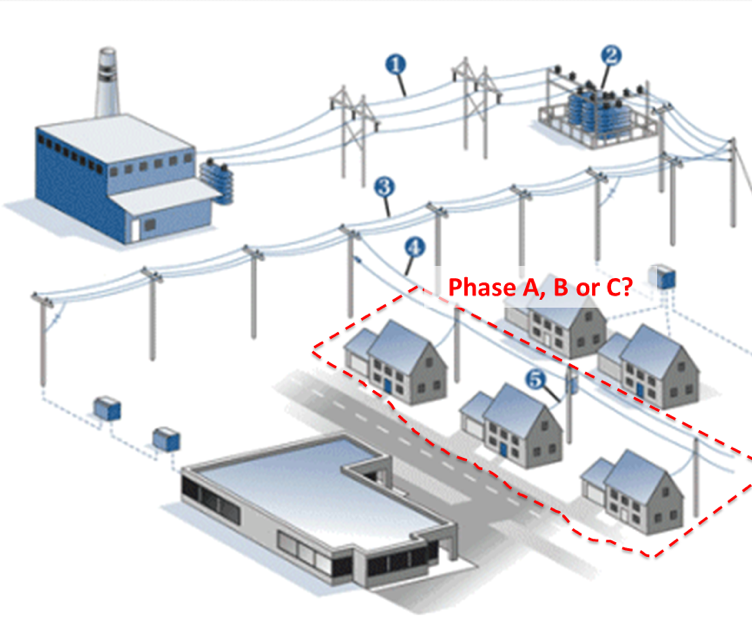
Modern distribution systems have been undergoing dramatic change in recent years. Increasing penetration of highly uncertain components (e.g. rooftop solar panels) poses great operational challenges to distribution system operators. The traditional phase-balancing problem is typically solved using the expected load profile for the upcoming period. A hidden assumption behind this is that the (daily) load patterns change at a slow rate, and uncertainties do not have a critical impact. For a modern distribution system with deep penetration of intermittent generation resources, this underlying assumption becomes unrealistic, since more temporal fluctuations and uncertainties appear in load profiles. As a consequence, the phase-balancing problem for modern distribution systems should be a real-time decision-making problem in an uncertain environment, in response to rapid and stochastic changes of load patterns.
The distribution grid has become a focal area of research given all the new technological innovations that are happening at the edge of the grid. It is not surprising that during the Fall 2018 Simons Institute program on Real-Time Decision Making, a group of researchers began to get together on a regular basis to look into this problem. Among the participants were Pravin Varaiya, Steven Low, Evdokia Nikolova, Swati Gupta, Dileep Kalathil, Le Xie, and several postdoctoral researchers and graduate students, including Ali Khodabakhsh, Xinbo Geng, Emmanouil (Manolis) Pountourakis, and Sivaranjani Seetharaman.
As an example that grew out of the discussions, we present below a robust look-ahead operation framework for phase balancing in modern distribution systems [1]. To deal with challenges from intertemporal changes of loads, we incorporate a rolling-window (model predictive control) type of operation. We use techniques from robust optimization to handle uncertainties in the loads. The proposed framework seeks balanced phases which are robust to all realizations of demand, in an uncertainty set learned from historical data.
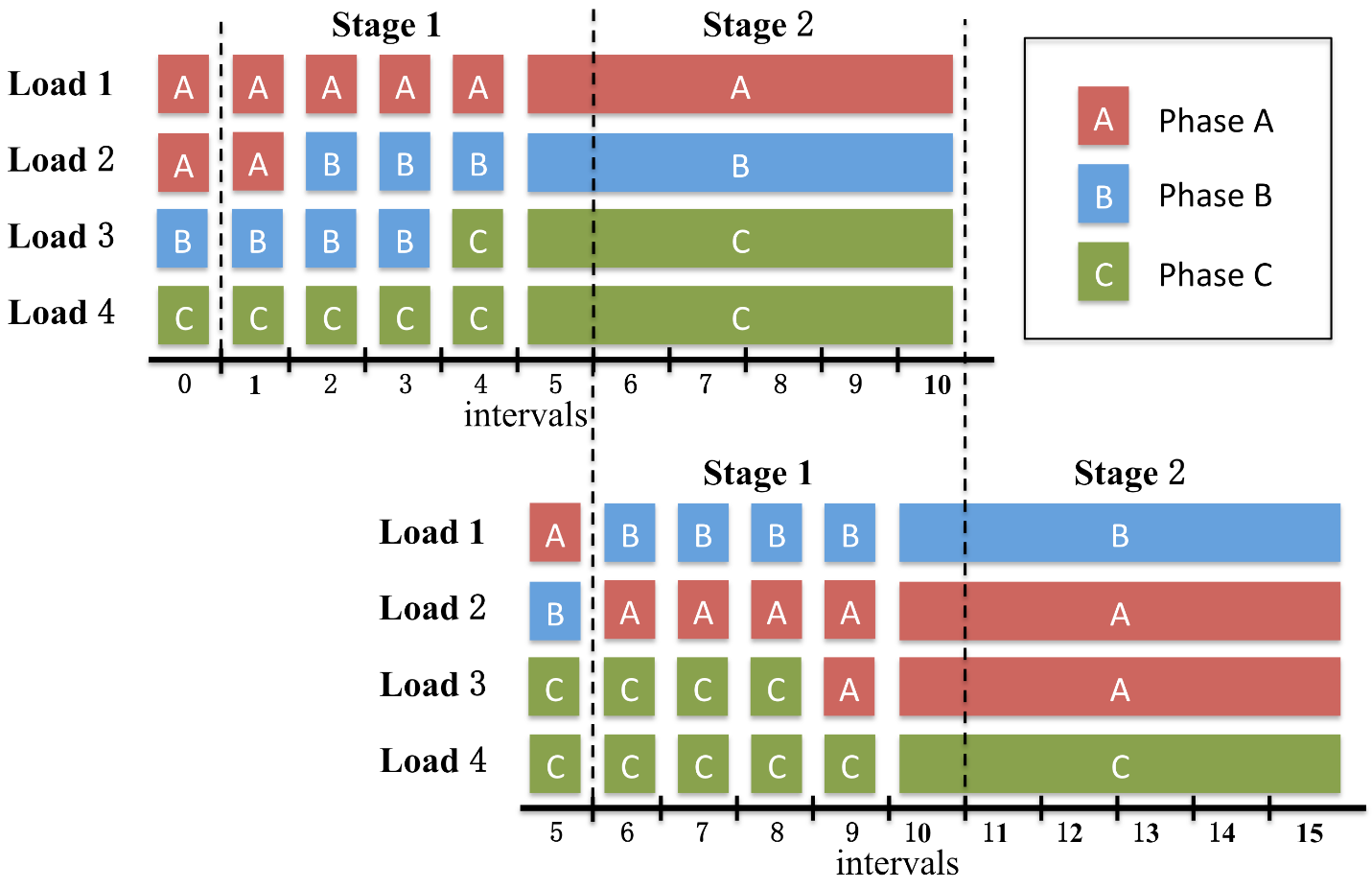
The phase-balancing problem is typically formulated as a mixed-integer linear program, which is computationally intractable in general. Currently, we are seeking approximation algorithms which can return high-quality solutions in a short time. Phase-balancing problems are related with the classical minimum-makespan scheduling problem, which attempts to run a given set of jobs on a fixed number of parallel machines such that total time (i.e. the makespan) to complete jobs on any machine is minimized. In current ongoing work, we are trying to find connections between a robust data-driven approach and approximation algorithms for the stochastic version of scheduling problems, to help generalize the model to incorporate switching costs.
Real-Time Localization of Forced Oscillation in Large Transmission Grids
Forced oscillation localization is a canonical problem in the power-system community. From a control perspective, the power grid can be characterized by a large-scale oscillatory system with multiple inputs and multiple outputs. An input of the system can vary periodically owing to a malfunctioning device. Then, such a periodical input drives the measurements over the grid to exhibit oscillatory behavior. These oscillations observed at measurements are termed as forced oscillations. Accordingly, the malfunctioning device associated with the periodic input is called the source of forced oscillations. The goal of the forced-oscillation localization problem is to pinpoint the measurements geographically closest to the oscillation source. Without timely localization of forced oscillations, small oscillations at the beginning can incur large oscillations over the power grid. The resulting pronounced oscillations may lead to uncontrollable cascading failure and large-scale blackouts, e.g. the Western North America electric blackout in 1996. Therefore, it is imperative to prepare system operators with an online decision-support tool for forced oscillation localization.
The discussion of an interconnected oscillatory system can be traced back to 1656, when coupled oscillations were characterized mathematically based on first principles by Christiaan Huygens. However, the immense complexity of a large-scale power system, exacerbated by a diverse generation portfolio and flexible demand, may render the classically physics-based analysis infeasible in the context of near real-time localization of forced oscillation. In view of such complexity in physics-based analysis for bulk power systems, we consider a novel perspective to interpret forced oscillations and achieve the localization in a data-driven yet physically interpretable fashion. We formulate the localization problem as separating low-rank components from high-dimensional synchrophasor data using Robust Principal Component Analysis (RPCA), which can be solved by the Augmented Lagrange Multiplier method [2, 3].
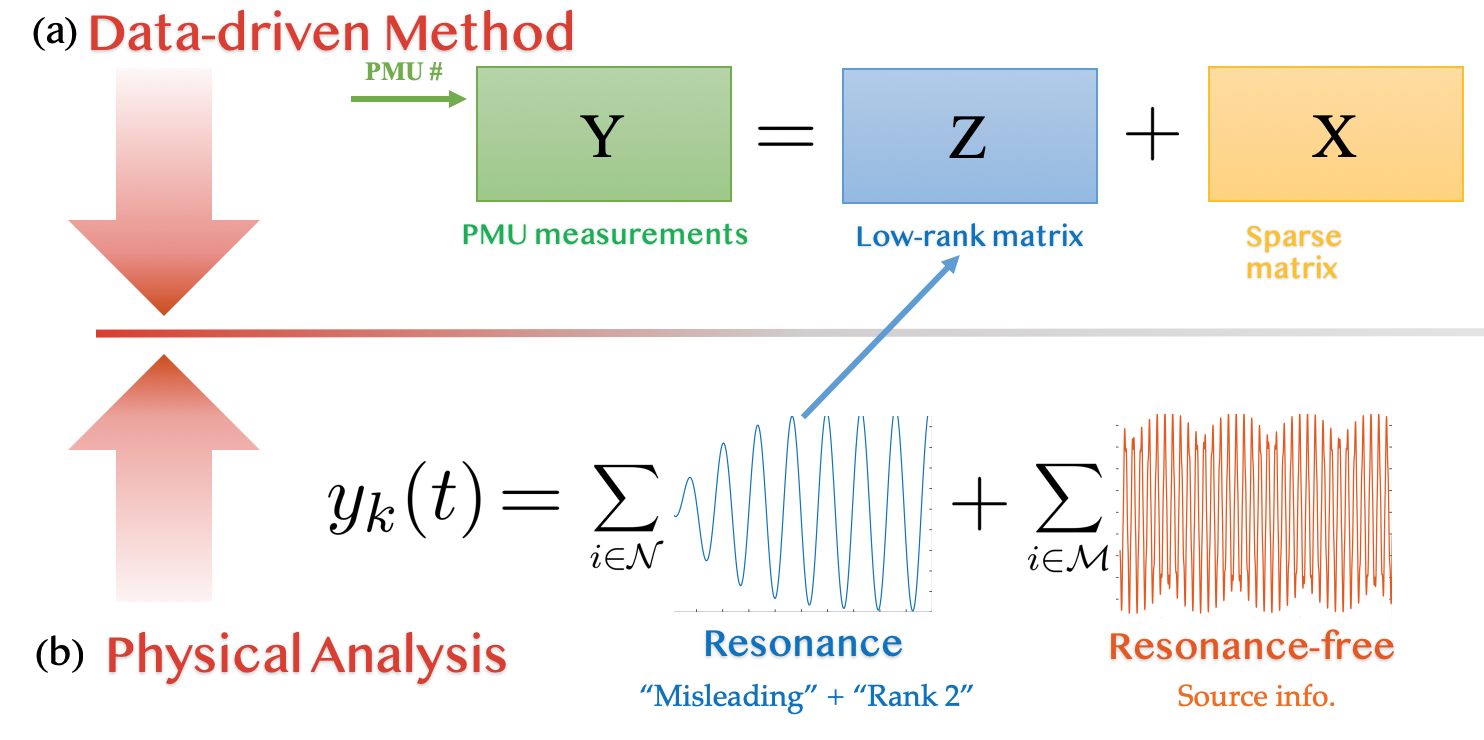
Nevertheless, given the high dimensionality of real-world measurement data in power systems, the scalability of the RPCA-based method is a predictable barrier to the method’s practical implementation. One promising direction is to design a distributed RPCA algorithm. The advanced data acquisition system in the power grid, i.e. the synchrophasor system, has a hierarchical architecture: measurements collected by individual synchrophasors are first streamed to the Phasor Data Concentrator (PDC) in their respective areas; the synchrophasor samples in each PDC are then transmitted to a control center. Compared with processing all synchrophasor measurements in the control center [2, 3], it is desirable to equip each PDC with a data pre-processing algorithm which can extract low-dimensional signatures for forced oscillation localization in each area. Such decentralized RPCA requires significant innovation on computation algorithms. Our ongoing work aims to design such a pre-processing algorithm for the purpose of decreasing the computational burden of the control center.
References
[1] Xinbo Geng, Swati Gupta, and Le Xie. “Robust Look-Ahead Three-Phase Balancing of Uncertain Distribution Loads,” Proceedings of the Hawaii International Conference on System Sciences, Jan 2019.
[2] Tong Huang, Nikolaos M. Freris, P. R. Kumar, and Le Xie. “Localization of forced oscillations in the power grid under resonance conditions.” In Information Sciences and Systems (CISS), 2018 52nd Annual Conference (IEEE, 2018): 1-5.
[3] Tong Huang, Nikolaos M. Freris, P. R. Kumar, and Le Xie. “Forced Oscillation Localization in Electric Power Systems under Resonance Conditions.” arXiv preprint arXiv:1812.06363 (2018).
[4] Steven H Low, “Convex Relaxation of Optimal Power Flow — Part I: Formulations and Equivalence.” IEEE Transactions on Control of Network Systems 1, no. 1 (2014): 15-27.